Introduction
Artificial intelligence (AI) and its applications of AI in agriculture are rapidly reshaping many industries, including the century-old field of agriculture. The intelligent integration of AI technologies stands to transform farming practices worldwide by optimizing productivity, sustainability, and farm management.
This article explores the strategic role of AI in agriculture to illustrate its potential as a foundational innovation. It examines prominent Use cases of AI in agriculture reshaping farming operations, quantifies statistics on AI adoption, identifies future opportunities, and discusses overcoming challenges to maximizing benefits safely.
Overall, the analysis underscores AI’s prominence for revolutionizing global food production through data-driven insights and automation that complement human endeavor. Understanding AI’s significance helps agriculture stakeholders harness its full capabilities to positively impact livelihoods for generations to come.
The Changing Face of Farming with AI
Traditional agriculture faces increasing demographic, climatic, and resource constraints that impact global food security. Leveraging AI addresses these pressures by streamlining processes through intelligent automation, remote sensing, and predictive insights. This elevation advances sustainable practices and maximizes yields facing uncertain futures.
Precision Farming Transforms with AI
Traditional blanket applications of AI in agriculture disregard variabilities across fields. AI-powered precision farming tailors inputs considering soil composition, temperature, moisture levels and more through sensors. AI leverages correlated data to determine optimal seeding densities, watering schedules, and pesticide distribution throughout each acre.
Real-time Soil Monitoring for Proactive Stewardship
Continuous automated soil analysis allows preemptive interventions. AI identifies lacking nutrients from electrical conductivity readings. It can predict yield reductions or advise supplementary applications long before visual symptoms appear, optimizing resource allocation.
Prediction Bolsters Planning and Resilience
AI forecasts weather, disease spread and more using vast historical data. Predictive insights help anticipate challenges, schedule operations, and safeguard profits against potential losses. Forecasts enable tactical adaptations versus reacting to surprises that risk returns.
Robotics Automate Mundane, Risky Tasks
Farm robots take over manual, repetitive, hazardous activities like weeding and harvesting that strain human labor pools. With RPA consulting services, autonomous bots are optimized to perform 24/7 without fatigue-induced errors or injuries from machinery. This enhances productivity and safety simultaneously.
Data-Driven Traceability and Compliance
Distributed ledgers record verifiable provenance data for regulatory compliance and brand protection. Smart contracts automate emission offset commitments. Transparent traceability builds consumer trust from seed to shelf.
Customized Management Plans
AI tailors precision recommendations to individual operations. Factoring complex on-farm variables, AI simultaneously balances yields with sustainability and profitability. Personalized stewardship enhances financial performance over broader technology adoption alone.
Predictive Maintenance Prevents Downtime
Continuous equipment health monitoring aids predictive maintenance. AI alerts farmers proactively to equipment issues, shortening repair times. Proactive servicing minimizes disruptions to cultivation schedules from unplanned outages.
This evolutionary arc shifting farming from reaction to prediction is just beginning, promising longer term impact of AI in business we have yet to envision. With each advance, agriculture’s transformation positions it to feed future generations sustainably through data-driven operations.
Key Stats on AI Adoption in Agriculture
The integration of artificial intelligence into agriculture is backed by compelling statistics that highlight its growing significance:
Market Growth: The global market for AI in agriculture is projected to reach USD 8.50 billion by 2031, growing at a compound annual growth rate (CAGR) of 22.4% from 2023.
Investment Trends: In a notable acquisition reflecting confidence in agricultural technology advancements, John Deere acquired Blue River Technology for $305 million. This acquisition underscores the importance of robotics in enhancing yield management through precise herbicide application.
Adoption Rates: A recent survey indicated that 80% of agribusinesses recognize the potential benefits of implementing AI solutions but only 20% have fully adopted these technologies. This gap presents significant opportunities for growth as more companies seek to leverage AI-driven insights.
AI Tools Advance in Fields: 85% of farmers using AI report its practical value for data collection through imaging/sensing. 77% saw benefits for improving resource allocation.
Predictive Analysis Gains Traction: 63% of agribusinesses surveyed by KPMG now use predictive analytics for assorted applications of AI in agriculture including yield forecasting.
AI Investment Climate Heats Up: Global corporate venture capital funding for agritech including AI rose 126% in 2022 to $6.7 billion according to AgFunder.
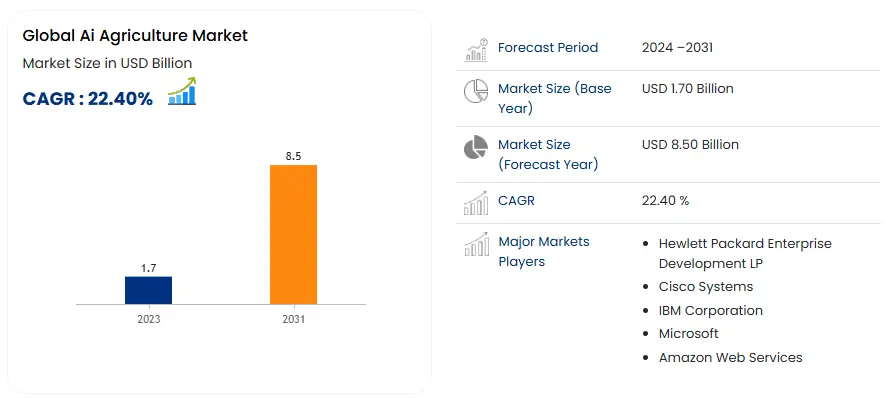
Such metrics underscore AI’s expanding presence across the agricultural industry as stakeholders increasingly embrace its potential. Continual innovation is driving further mainstreaming alongside rising demand for agricultural transformation.
Use Cases of AI in Agriculture
A dynamic range of Use cases of AI in agriculture are enhancing agricultural operations and processes through data-driven precision. This section provides an overview of AI’s strategic role across the agriculture value chain:
Precision Farming
AI-powered precision agriculture automates field-level crop management. Advanced soil analytics and yield forecasting optimize resource allocation. AI guides variable rate application of seeds, fertilizers, water and chemicals. Automated weeding helps reduce herbicide use and labor. AI and IoT enhance efficiency and sustainability.
Case Study: Farmers Edge
Farmers Edge leverages AI and IoT through its SST platform. It captures granular field data from satellites and sensors to generate prescriptive insights. Automated equipment then executes variable rate applications per AI guidance. This precision approach drives higher, more consistent yields while minimizing waste. Significant operational and environmental benefits result.
Livestock Monitoring
Precision livestock focuses on herd health and productivity. IoT technologies including vision systems, RFID, and collar-worn monitors provide 24/7 animal surveillance. AI derives insights from sensor recordings, informing targeted interventions. Automation improves outcomes while mitigating labor constraints.
Case Study: Cainthus
Cainthus deploys computer vision and IoT leveraging on-farm cameras to monitor cattle behavior. Its AI detects abnormal signs early, alerting farmers to potential issues. Analysis determines root causes to guide remedial action. Automated health surveillance and predictive diagnostics optimize outcomes while respecting animal welfare. Data-driven insights streamline livestock management.
Crop and Soil Monitoring
Advances in remote field evaluation enable detailed crop and soil analytics anywhere. Sensors integrated into drones and farm machinery continually collect real-time data on vital crop characteristics. Computer vision examines fields and fields for nutritional deficiencies, pests or diseases. AI assures sustainable production through informed response.
Case Study: Agerpoint
Agerpoint develops AI-guided sensor systems for precision agriculture including crop and soil analysis. Drones scan fields to detect deviations from optimal vigor and generate prescription maps. On-combine sensors identify nutrient deficiencies. AI analyzes spectral and visual measurements, identifying issues for targeted treatment. Timely interventions maximize yields sustainably.
Pest and Disease Detection
Early identification prevents crop losses from pests and diseases. Computer vision examines plant tissues for anomalies indicative of infestations. Deep learning algorithms recognize symptomatic features to accurately diagnose issues. Alerting farmers to containment needs expedites treatment timing for improved efficacy.
Case Study: BAIDU
BAIDU developed an AI-based pest detection system leveraging computer vision. Algorithms analyze high-resolution images of cotton plants to recognize pests like the cotton bollworm. The AI model determines infestation severity levels with over 90% accuracy. Precision identification helps farmers apply precisely targeted control measures against identified parasites, reducing costs and waste compared to broadcast spraying.
Predictive Analytics for Farm Management
AI excels in big data pattern recognition, enabling predictive insights from vast datasets. By analyzing climate records, soil conditions and historical yields, algorithms foresee optimal sowing windows, planting densities and varietal choices. Recommendations harmonize requirements across fields, maximizing crop production sustainably farm-wide.
Case Study: CropIn
CropIn uses AI and IoT to offer predictive agricultural intelligence worldwide. By analyzing satellite imagery, weather patterns, and field-specific data, algorithms forecast yields and advise optimized practices for various crops. Recommendations optimize seed selection, nutrient applications, irrigation schedules and more per precise field characteristics. Outcomes include reduced costs and increased, more consistent outputs through precision farming powered by AI.
Automated Irrigation Systems
Computerized irrigation guided by AI monitors soil moisture levels against plant needs and the water table. Sensors transmit real-time data on environmental factors. AI decides water amounts and schedules tailored to specific sections. Automated systems conserve water usage while fulfilling crop hydration requirements.
Case Study: Agricultural Improvement Company
AIC developed SuperFlo irrigation controllers utilizing AI and computer vision. Sensors integrated into Center Pivot irrigation systems capture imaging and metrics of soil conditions. AI analyzes this data against crop water demands to autonomously adjust water distribution. SuperFlo optimizes water usage by halving applications yet increasing yields up to 30% through precision. Significant cost savings and output gains result.
Agricultural Robotics
Farm automation reduces drudgery through robots that execute physical agricultural tasks. Agricultural drones aid crop dusting, spraying and scouting. Robotic milking parlors handle milking freeing farmers for other duties. Harvesting robots cut, collect and pack produce. Weeding and planting robots precisely handle crops. AI coordinates robot fleets through computer vision, localization and path planning.
Case Study: Agrobot
Agrobot developed autonomous strawberry harvesting robots using AI and machine vision. Robotic arms and cameras identify ripe berries. AI algorithms direct precise fruit picking and placement into containers. Agrobot robots harvest 50% faster than manual labor while being gentle enough not to damage fruits. Computer vision enables 95% pick rates with minimal human involvement needed. Robots upgrade harvest efficiency.
Digital Farm Management
Precision farming tools digitally connect field data for optimized oversight. AI assimilates contextual data including satellite imagery, irrigation systems, tractor sensors into a unified digital model. Farm managers gain visibility into the “digital twin”, accessing insights into crop conditions, soil properties and implications for optimum field practices.
Case Study: Agerpoint
Israeli AgTech Solutions startup Agerpoint provides an AI-powered digital farm management platform. Their platform incorporates data from sources like aerial and drone images, soil moisture sensors and active farm equipment tracking via machine vision. Algorithms analyze this assortment of field data to build highly granular digital field maps. Farm managers access these field layering models on desktop or mobile for real-time field overviews and precision management decisions.
Logistics and Supply Chain Optimization
Blockchain and AI streamline agricultural logistics by improving traceability, automating transactions and optimizing transport and storage routes through predictive analytics. Smart contracts enable seamless exchange of goods and settlement without intermediaries. Distributed ledgers bring transparency through immutable records across fragmented global agricultural supply networks.
Case Study: IBM Food Trust
IBM developed the blockchain-powered Food Trust network to bring transparency across the food supply chain. The solution digitally traces produce from origin to store shelves using distributed ledgers. Participating growers, processors, distributors, importers and retailers record production, handling and transport events on the network. Buyers access detailed supply chain histories through a mobile app to enhance food safety. Governments leverage the platform’s immutable audit trail for regulatory oversight.
Predictive Analytics and Market Insights
AI algorithms analyze petabytes of external and proprietary data to generate prescient market intelligence. AI predicts commodity price fluctuations and assesses factors impactingagribusiness performance. Insightsinto outcomes from proposed strategies aid strategic optimizations. AI surfaces untapped new market opportunities increasing profitability through advanced data analytics.
Case Study: Descartes Labs
Descartes Labs applies AI and big data analytics to extract market insights from petabytes of geospatial, financial and climate data in the agricultural sector. Custom algorithms predict yields, assess weather impacts and gauge commodity price projections. Insights help farms optimize resource allocation, enhance risk management and make data-driven operational and strategic decisions. Clients access detailed forecasts and recommendations powering informed decision making across supply chains.
Agricultural Drones
Routine farming tasks are streamlined using AI-powered agricultural drones with advanced peripherals like sensors, hyperspectral cameras and RGB cameras. Computer vision analyzes imagery for early identification of issues impacting production including nutrient deficiencies, water stress, diseases and infestations. Autonomous spraying ensures accurate, judicious and safe application of inputs.
Case Study: AgEagle Aerial Systems
AgEagle develops full-stack drone solutions for precision agriculture. Their drones equipped with 4K sensors, computer vision and AI conduct field analysis, irrigation monitoring and crop spraying. AI processes aerial imagery to identify issues impacting crop growth. Spraying robots controlled by AI apply chemicals while precisely targeting only problem areas based on computer vision guidance. This precision eliminates wastage and reduces environmental impact.
Digital Farm Management Systems
Management dashboards equipped with AI aggregates data from fields, drones, equipment, IoT sensors and weather stations into an integrated view. AI-powered insights inform strategic decision making. AI correlates temporal and spatial datasets to detect yield-impacting patterns and formulate data-driven recommendations.
Case Study: CropX
Canadian agriculture technology firm CropX developed AI-powered farm management software that connects disparate data sources. Their AI-driven analytics platform collects real-time field information alongside historical yields and sensor data. Integrated AI models analyze the amalgamated dataset to provide predictive insights regarding optimal field conditions, resource allocation and yield forecasts. The AI-powered digital farm model enhances operational optimization and informs strategic planning.
This concludes an exploration of impactful AI applications transforming agriculture through advanced data-driven insights and process automation. These diverse Use cases of AI in agriculture demonstrate AI’s growing relevance in revolutionizing the agricultural landscape through precision, sustainability and optimized decision-making. Further innovation and integration of these technologies within agricultural practices portends enhanced productivity, resilience and economic opportunity in the years ahead.
Advantages of AI Applications in Agriculture
The wide-ranging opportunities that artificial intelligence presents to agriculture signals its strategic significance as a foundational technology revolutionizing this industry. Beyond specific Use cases of AI in agriculture, AI delivers noteworthy advantages that enhance sustainability, productivity and profitability enterprise-wide. Some prime benefits include:
24/7 Monitoring for Prompt Issue Detection
AI platforms seamlessly survey fields, barns and pastures around the clock via surveillance cameras, drones, IoT sensors and other tools. Constant observation allows early identification of potential issues before major impacts materialize, facilitating timely response.
AI spots sickness symptoms, pest infestations, injuries or other abnormalities that humans may overlook or identify too late for optimal intervention. Continuous animal monitoring alleviates labor demands, while supporting optimal herd health.
Real-Time Adaptability to Environmental Changes
AI gleans insights from live data streams, such as sensor feeds and imagery, to continuously refresh insights. This real-time situational awareness supports prompt adaptations to changing conditions through data-driven recommendations.
AI accommodates dynamic risks posed by fluctuating weather, emerging pests or evolving markets more capably than rigid protocols. Continuous updates empower timely pivots when anomalies emerge.
Personalized Insights and Recommendations
AI profiles individual fields, farms and livestock based on multivariate datasets. Its personalized insights allow precision-focused management tailored to each unique agricultural system.
AI considers localized soil compositions, weather patterns, and historical yields alongside existing infrastructure when crafting guidance. AI personalization optimizes resource allocation and management approach at micro levels.
Automation and Streamlining of Manual Tasks
Repetitive procedures like irrigation programming, scouting, and demand forecasting demand labor but offer little value-add. AI automates such duties, freeing personnel for strategic priorities.
AI oversees tasks autonomously according to programmed parameters, maintaining preset schedules and responding immediately to triggers. Automated workflows optimize resource allotment across farming operations.
Usage of Alternative and Environmental Data
AI in Agricultural Industry integrates previously untapped data sources like satellite imagery and soil moisture readings into analyses. This expands insight beyond traditional inputs to include remote sensing, climate, and spatially-dependent factors.
The incorporation of alternative data sources enhances precision across AI in Agricultural Industry processes by factoring spatial context lacking from legacy methods reliant solely upon fixed measurements.
Quantified Projections and Predictive Capabilities
AI in Agricultural Industry numbers future climate conditions, crop yields, pest prevalence probabilities and market price fluctuations based on analyzing massive historical datasets. Generated forecasts provide numerical visibility into forthcoming financial outcomes and operational impacts.
Quantified projections empower strategic preparation well in advance – enabling proactive adjustments and mitigation of projected risks. Predictive guidance strengthens planning across crops and commodity classes.
Potential Timesaving and Cost Reductions
Robotic equipment assisted by AI executes farm tasks around the clock with reduced input requirements compared to manual tasks. AI also streamlines processes like compliance monitoring and field analytics that traditionally drain timetables.
Integrating AI in Agricultural Industry can potentially automate repetitive duties, shedding labor costs while freeing workers towards higher-impact roles. Over time, this time-saving element of AI lowers operational overhead substantially.
Collectively, these AI-enabled advantages confer meaningful competitive differentiators for agricultural enterprises that pursue its adoption. From enhanced resilience against macro challenges to optimized production outcomes – the strategic rewards serve as catalysts for sustained business performance in a technology-driven agricultural future.
Challenges of Implementing AI in Agriculture
While artificial intelligence presents numerous benefits within agriculture; several challenges impede its widespread adoption:
Lack Of Understanding Of AI Tools: Many farmers remain unfamiliar concerning how best utilize available technological resources effectively; leading toward underutilization despite potential advantages offered through implementation strategies tailored specifically toward enhancing operational efficiencies observed regularly throughout diverse agricultural settings.
Slow Adoption Rates Within The Sector: Despite recognizing potential benefits associated with implementing innovative solutions; many agribusinesses exhibit hesitance due primarily towards perceived costs involved alongside uncertainties surrounding return-on-investment calculations performed prior initiating adoption processes effectively over time.
Familiarity With Traditional Methods: Resistance towards adopting new methodologies stems largely from long-standing reliance upon traditional practices ingrained deeply within cultural norms observed across various regions globally; ultimately hindering progress toward modernization efforts aimed at improving overall productivity levels achieved consistently over time.
Communication Gaps Among Stakeholders: Effective collaboration between different stakeholders—including tech developers alongside end-users—is critical towards ensuring successful implementation outcomes achieved consistently throughout diverse agricultural settings; however communication gaps often arise leading toward misunderstandings impeding progress made towards achieving desired objectives effectively over time.
Awareness And Education Regarding Available Solutions: Limited knowledge concerning available technological options hinders decision-making processes among many small-scale producers who may lack access to necessary resources required understanding complexities involved surrounding modernizing operations effectively over time.
Why Choose A3Logics as Your AI Software Development Company?
Choosing A3Logics as your preferred partner for developing customized software solutions, including as an enterprise AI development company, tailored specifically towards enhancing agricultural operations offers numerous advantages:
- Expertise In Agricultural Domain: A3Logics possesses extensive experience working within AgTech Solutions sectors; enabling them deliver high-quality products aligned closely with industry standards observed regularly throughout diverse operational contexts effectively over time.
- Comprehensive Service Offerings: From enterprise AI chatbot development services focused primarily upon creating top applications of AI capable of managing complex datasets generated during farming activities through chatbot development aimed facilitating communication between stakeholders involved—all aspects covered comprehensively ensuring seamless integration achieved consistently throughout diverse agricultural settings effectively over time.
- Commitment Towards Sustainability Initiatives: A3Logics prioritizes environmentally conscious methodologies aimed at promoting sustainable practices within the agriculture sector; ultimately contributing positively toward addressing pressing global challenges faced today including food security concerns arising amidst rapidly changing climatic conditions encountered regularly globally.
Final Take
The significance of artificial intelligence in the agriculture industry cannot be overstated as it paves the way for a more efficient, productive, and sustainable agriculture in farming practices worldwide. By harnessing advanced technologies such as predictive analytics and automation solutions powered by machine learning algorithms; farmers gain valuable insights enabling them optimize operations while conserving vital resources essential towards meeting growing food demands faced globally today amidst rising population pressures encountered regularly across diverse regions globally. So, connect with one of the top AI Development Companies named A3Logics and get all the assistance you need.
Transform Your Agricultural Software Vision into Reality – Book A 30 Minutes Free Consultation!
FAQs
1. How does AI assist in sustainable farming practices?
Artificial intelligence in agriculture significantly contributes to sustainable farming practices by optimizing resource use and minimizing environmental impact. AI technologies enable precision agriculture, allowing farmers to apply water, fertilizers, and pesticides only where needed, reducing waste and chemical runoff. For instance, AI-driven soil sensors monitor moisture levels and nutrient content, helping farmers make informed decisions about irrigation and fertilization. Additionally, AI Development Services can analyze weather patterns and predict pest outbreaks, enabling proactive management that reduces the need for chemical interventions. By promoting efficient resource management and reducing reliance on harmful chemicals, AI supports sustainable farming practices that are essential for long-term agricultural viability.
2. Can Artificial intelligence in agriculture improve water management in agriculture?
Yes, AI can greatly enhance water management in agriculture through precision irrigation systems that utilize real-time data to optimize water usage. By employing sensors and machine learning algorithms, AI can assess soil moisture levels and weather conditions to determine the precise amount of water needed for crops at any given time. This targeted approach minimizes water wastage and ensures that crops receive adequate hydration without overwatering. Furthermore, AI can help identify leaks or inefficiencies in irrigation systems, allowing for timely repairs and maintenance. Overall, AI-driven water management solutions contribute to more sustainable agricultural practices by conserving vital water resources.
3. How does AI support agricultural equipment maintenance?
AI supports agricultural equipment maintenance through predictive analytics that monitor machinery performance in real-time. By analyzing data from sensors installed on equipment, AI can identify patterns and detect anomalies that may indicate potential failures before they occur. This proactive approach allows farmers to schedule maintenance activities at optimal times, reducing the risk of unexpected breakdowns and costly downtime during critical farming operations. Additionally, Artificial intelligence in agriculture can provide insights into the optimal usage of machinery, helping farmers extend the lifespan of their equipment while improving overall operational efficiency.
4. Can Agricultural AI solutions enhance the marketing approach?
Absolutely! Agricultural AI solutions enhance the marketing of agricultural products by analyzing consumer behavior and market trends to inform pricing strategies and promotional efforts. Machine learning algorithms can process vast amounts of data from various sources—such as social media, sales records, and market reports—to identify emerging trends and consumer preferences. This information enables farmers and agribusinesses to tailor their marketing strategies effectively, ensuring that products meet consumer demands while maximizing profitability. Additionally, AI-driven tools can optimize supply chain logistics by predicting demand fluctuations, ensuring that products reach consumers promptly while minimizing waste.
5. What is the impact of Agricultural AI solutions on industry?
The impact of AI on agriculture is profound and multifaceted. By enhancing productivity through precision farming techniques, farmers can achieve higher yields while using fewer resources such as water and fertilizers. This efficiency not only boosts profitability but also contributes to environmental sustainability by reducing waste and chemical usage. Furthermore, AI facilitates better decision-making through predictive analytics that help farmers anticipate challenges related to weather, pests, and market demands. Ultimately, the integration of Agricultural AI solutions leads to more resilient farming systems capable of adapting to changing conditions while ensuring food security for a growing global population.