Artificial intelligence is revolutionizing the supply chain by introducing advanced levels of automation, predictive insights, and optimization to traditional management processes. Where manual oversight once reigned, AI brings transformative potential through machine autonomy bolstered by vast historical data.
This evolution stems from AI’s capacity for sophisticated pattern analysis, continuous learning from massive datasets, and subsequent ability to generate valuable recommendations. By mining vast volumes of structured and unstructured information, AI delivers predictive visibility and strategic guidance that amplifies human decision-making within supply chain operations.
This article provides a comprehensive overview of AI’s strategic role within supply chain management. It examines prominent Use cases of AI in Supply Chain demonstrating AI’s real-world applications and benefits. Furthermore, insights into both achievements and current challenges illustrate AI’s transformative trajectory within this critical business function.
The analysis aims to underscore AI’s strategic importance while outlining a pragmatic roadmap for leveraging its intelligence effectively. With a balanced perspective, the discussion underscores both opportunities and responsibilities surrounding AI’s integration into global supply chains.
Understanding the Role of AI in Supply Chain Management
AI is playing a vital role in optimizing supply chain operations and management. Supply chain management involves planning, sourcing, manufacturing, delivering and returning of products efficiently. It encompasses coordination between suppliers, manufacturers, warehouses and retailers. With globalization and rising customer expectations, managing supply chains has become more complex. This is where AI comes into the picture by helping analyze huge volumes of data, gain insights, identify patterns and make predictive decisions.
Some key roles played by AI in supply chain management include demand forecasting and planning, inventory optimization, predictive analytics, automated decision making, preventing shipment delays, reducing costs, improving customer experience, supporting sustainability initiatives, enabling real-time visibility etc.
AI also helps enhance collaboration between suppliers, facilitates knowledge sharing and improves communications. In essence, when coupled with technologies like machine learning, AI acts as an intelligent brain that streamlines processes, improves efficiency of AI in Supply Chain and helps companies gain a competitive edge.
AI in Supply Chain: Stats and Facts
The impact of AI on the supply chain industry is supported by compelling statistics that highlight its transformative potential:
- Market Growth: According to a report by Market Research Future, the AI in Supply Chain market is expected to grow significantly over the coming years due to increasing demand for automation and efficiency across industries.
- Cost Reduction: A McKinsey study found that early adopters of AI-enabled supply chain management have reduced logistics costs by 15%, improved inventory levels by 35%, and enhanced service levels by 65%.
- Increased Efficiency: Gartner reports that organizations implementing AI technologies in their supply chains have seen improvements in operational efficiency by up to 30%.
- Demand Forecasting Accuracy: Companies using AI for demand forecasting have reported accuracy improvements of up to 50%, allowing them to optimize inventory levels effectively.
- Enhanced Decision-Making: A survey conducted by IBM indicated that 80% of executives believe that AI will significantly improve decision-making capabilities within their organizations.
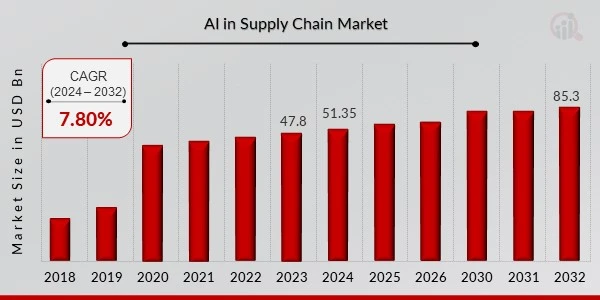
Top 20 Use cases of AI in Supply Chain
AI is finding a wide range of applications across the supply chain landscape. From demand forecasting and inventory optimization to warehouse automation and supplier relationship management, AI is delivering value at each link of the supply chain network. Here are some of the top 20 Use cases of AI in Supply Chain that are helping transform supply chain operations of various industries.
Back-office automation
One important use case of AI in the supply chain is automating back-office and repetitive tasks through technologies like robotic process automation (RPA). For example, tasks like data entry, document processing, invoice management etc. which are rule-based and involve large volumes of structured data can be automated. This frees up the time of back-office staff to focus on high value tasks. A real-world example is DHL, the logistics company using AI-powered digital workers for tasks like invoice processing and data migration during an M&A project. It helped achieve cost savings, process standardization and 70% reduction in turnaround times.
Logistics automation
AI is fueling innovation in transportation and logistics through technologies like autonomous vehicles. For example, companies like A3Logics are developing self-driving trucks for cargo transportation. Startups like Embark and TuSimple have also successfully tested autonomous trucks on public roads carrying real cargo. AI is also being used for routing and scheduling optimization. AI Development Companies like A3Logics help their logistic clients optimize fleet utilization, reduce empty miles and find the most fuel-efficient routes using machine learning models. This helps lower operational costs and carbon footprint.
Warehouse automation
Intelligent automation solutions powered by computer vision, robots, autonomous mobile robots and AI are revolutionizing warehouse operations. AI allows machines to see, understand their environment, navigate autonomously as well as collaborate with humans in dynamic workflows. For example, Amazon uses Kiva robots for moving goods, Putaway robots for storage and Drive units to transport carts in warehouses. Walmart has deployed autonomous picking carts called Alphabots in its warehouses. Such implementations have led to increased productivity, reduced costs as well as enhanced safety of warehouse employees.
Demand forecasting and planning
AI and machine learning are increasingly used for demand forecasting which is crucial for supply chain planning. Algorithms can consider a variety of factors like seasonality, pricing, customer demographics etc and predict demand more accurately compared to traditional techniques. For example, A3Logics helps retail clients forecast demand at a hyper-local level to effectively plan inventory. US food giant Mondelez leverages AI to improve demand forecasting for key brands like Oreo cookies by 10%. Accurate forecasts help optimize inventory levels and meet customer demands.
Inventory optimization
AI helps develop optimized inventory carrying policies to lower costs while maintaining high service levels. Algorithms analyze historical inventory and demand data to determine optimal stock levels of items based on attributes like lead times, shelf life etc. They can also recommend re-order quantities and safety stocks. For example, consumer goods giant P&G uses AI to closely match regional demands, avoid stock-outs as well as reduce obsolete inventory worth millions annually. AI tools from Inventory Management in Supply Chain Companies optimize a delicate cost-service tradeoff.
Predictive analytics/forecasting
AI exhibits human-like reasoning abilities to generate insights and make predictive forecasts. It analyzes massive internal and external data sets to identify relationships, patterns and anomalies that humans may miss. Retail giant Walmart leverages AI to generate thousands of micro-level sales forecasts daily for each product in every store. This is helping the company offer 30-40% better in-stock levels. AI and big data driven predictions help companies better plan resources, mitigate risks, take pre-emptive actions as well as improve operational and business outcomes.
Preventing Cargo threats
AI and machine learning are being used extensively to enhance cargo security through applications such as image recognition, natural language processing and anomaly detection. For example, AI enabled cameras and image recognition algorithms are deployed at ports to flag irregularities, detect stowaways or weapons. Companies are training ML models on massive historical data sets to identify suspicious vessels, cargo details or shipments at risk. This is helping authorities gain proactive intelligence, Profile high risk shipments and counter security threats.
Avoid Shipment delays
AI helps prevent delays through predictive routing and dynamic re-routing capabilities. Algorithms consider real-time variables like changing weather, traffic conditions, road closures to anticipate and route around potential delays. For example, project44 leverages AI in its global logistics platform. It helps shippers track in real-time, predict arrival times more accurately (within 15 minutes!) and notify about expected delays. This ensures on-time deliveries and better customer experiences for logistics providers and their clients.
Selecting and managing suppliers
AI enables data-driven supplier selection based on analytics of past performance, risks, costs, quality, production capacities and future potential. It also powers dynamic pricing recommendations, two-way collaboration and smart deal negotiation abilities. For instance, A3Logics helps brands select suppliers transparently based on criteria like ethical sourcing, sustainability. Its AI tool recommends best matching suppliers, negotiates deals and tracks full order lifecycles. This results in optimized profitability, high service and ethical sourcing.
Load Planning
Use of AI can optimize load planning – deciding what freight goes on each trailer, container, or other transport vessel. A3Logics’s AI tools help clients generate thousands of optimized load configurations daily based on weight limits and dimensions of vehicles and goods. This minimizes unused space, avoids overweight loads and reduces the number of shipments needed. Further, algorithms power dynamic rearrangements if new orders arrive. Proper load planning alleviates risks, reduces transportation costs while improving asset utilization.
Explore AI Solutions for Your Supply Chain!
Streamlining inbound logistics
AI plays a key role in inbound logistics through transport management systems and predictive ETA features. For example, project44’s AI-powered telematics control tower gives manufacturers a real-time view of raw material shipments. It predicts delays, tracks anomalies and helps proactively manage exceptions. This ensures smooth material flows into plants, avoids production disruptions and helps companies maintain high service levels with suppliers and OEMs. AI-driven visibility across multi-modal inbound logistics streamlines processes.
Improved Quality control
Computer vision and AI are helping automate quality inspection processes. Algorithms can detect defects invisible to human eyes by analyzing visual attributes like color, dimensions, weight distributions etc. For instance, A3Logics provides AI solutions to detect cracks in infrastructure, certify organic produce and flag counterfeits. Their AI tools achieve 95% accuracy, reduce false rejections and help companies pass only quality goods downstream. This enhances productivity, food safety as well as customer satisfaction.
Equipment maintenance
AI achieves smarter maintenance of logistics assets like vehicles, air conditioners, cranes through condition monitoring and predictive maintenance. Sensors generate telemetry that AI analyzes to forecast component failures. For example, AI from A3Logics helps predict truck breakdowns weeks in advance. This enables planned servicing, avoids unplanned downtime of equipment and eliminates revenue losses. By reducing maintenance costs up to 30%, AI is transforming uptime reliability across Industries that rely on mechanized handling equipment.
Enhanced customer service
AI powers intelligent virtual agents or chatbots that can field customer queries round the clock through natural conversations. They alleviate live agent loads, provide 24/7 support and personalized experiences. For example, A3Logics’s Claude AI chatbot fields logistics queries for DHL Express. It helps track shipments, provides updates on customs clearance and ensures smooth trade experiences. AI chatbots are enhancing customer satisfaction scores for global logistics providers as they address common pain-points effectively.
Bullwhip effect prevention
The bullwhip effect arises due to demand and supply fluctuations propagating upstream in a supply chain. This leads to overproduction, stockouts and higher costs. AI analyzes POS and inventory data from retailers to proactively smooth demand signals to manufacturers and mitigate oscillations. When integrated with Sales and Operations Planning tools, AI can break information asymmetries in supply networks, synchronize partners and reduce risks of disruptions from bullwhip effects. This adds resilience to supply chains.
Supporting sustainability initiatives
AI driven predictive analysis helps achieve better environmental sustainability. For example, AI developed by A3Logics helps clients optimize logistics routing to reduce distances traveled. Its visibility suite tracks real-time fuel consumption as well. This increases fleet efficiency up to 25% by avoiding empty miles and choosing green routes. AI also powers monitoring of effluents and resources in industrial facilities to flag anomalies and recommend optimizations. In essence, AI is playing a vital role in helping companies across Industries lower their carbon footprint.
Improved supplier communications
AI strengthens collaboration between suppliers and customers through virtual assistants and knowledge marketplaces. Chatbots address suppliers’ pain-points by automating activities like order tracking, payment reconciliations and inventory updates. AI chatbots provide personalized support and access to domain knowledge 24/7 without language barriers. This drives efficiencies, resolves issues faster and improves supplier relationships by reducing cycle times for transactions. Virtually managed supply partnerships powered by AI deliver better outcomes for businesses.
Helping ensure employee safety
AI and computer vision are being used to monitor employee safety in hazardous logistics, manufacturing and construction work environments. For example, A3Logics provides real-time monitoring solutions equipped with AI cameras to flag PPE violations, near-misses and risky behaviors. By analyzing visual feeds for attributes like distance between persons, head gear etc, AI alerts managers to intervene before actual safety incidents occur. Companies are deploying these tools to protect workers as well as comply with government regulations.
Automated inventory management
AI powers fully automated inventory verification and cycle counting processes through computer vision, sensors and IoT. Solutions from A3Logics and other AI Development Companies leverage machine learning algorithms and edge devices to continuously audit on shelf-availability and stock levels. This not only improves visibility but also avoids costlier physical audits. Fully automated inventory validation with AI results in higher process efficiencies, better fill rates, reduced risk of stockouts as well as improved cash flows for organizations.
Fraud Prevention and Detection
AI and machine learning are helping identify fraud patterns in supply chains and eliminate practices like counterfeiting. Algorithms process multi-channel transactional records, supplier details as well as news/social reports to flag suspicious trails and anomalies. They can analyze images to certify authenticity of goods as well. For example, AI tools from A3Logics authenticate medicines and eliminate fakes worth millions annually. AI models also flag supply chain risks continuously to help businesses deter gray markets and losses.
Benefits of AI in Supply Chain
Implementation of AI provides significant benefits across processes and functions of modern supply chains. From reducing costs and improving efficiencies to enhancing sustainability of operations and customer experiences, AI delivers tangible value in a relatively short payback period. Here are some top benefits of leveraging AI in supply chain management:
Lower operating costs
AI drives efficiencies that translate to considerable reductions in operating costs for supply chain organizations. Automating manual tasks improves productivity while optimizing processes eliminates non-value add activities. AI further helps make assets and resources highly utilized through perfect scheduling and demand-driven replenishments. Integration of AI Planning across production, inventory and transportation management results in less leftover inventory and redundant shipments. Overall, cost savings from implementing AI range between 10-30% depending on specific use cases. Logistics leader DHL for instance reported 15-20% reductions due to AI route optimization.
Advanced real-time decisions
AI algorithms analyze enormous incoming data in real-time to power predictive and autonomous decision making. By having visibility into demand signals, fulfillment status as well as stochastic foresights that humans may miss, AI tools can take pre-emptive actions without human interventions. For example, predicting potential shortages days in advance helps companies proactively make alternate arrangements to avoid stock-outs than reacting post fact. Such self-driving changes by AI systems are delivering step improvements over conventional periodic planning approaches. Real-time decisions also mitigate risks and uncertainties throughout end-to-end supply chain cycles.
Cut down on errors and waste
AI solutions improve quality and consistency by identifying anomalies on extensive datasets to root cause issues unattainable otherwise. Computer vision pinpoints defects invisible to humans while NLP detects nuanced communication misunderstandings across supply chain communities. For example, identifying assembly line errors leads production supervisors to correct issues before impacting volumes of outputs. In warehouse applications, AI prevents accidental movements like packing wrong items together. Eliminating inefficiencies, AI reduces costs through controlling unnecessary expenditures on fixing past mistakes and scrapping bad inventory. Businesses save millions through quality enhancement and waste reduction.
More tailored inventory management
Machine learning on demand forecasting allows predictive adjustment of stock levels per location, customer and item attributes to ensure right amounts at optimum costs. AI determines re-order quantities so as to avoid stock-outs as well as carry fewer unneeded units incurring storage expenses. This tailoring enhances fill rates for customers while freeing up working capital locked in excess stock for alternative use. AI’s ability to forecast personalized needs and respond granularly across varied business segments delivers another 10-30% improvement in inventory turns and shrinkages. Overall it transforms inventory performance through hyper-targeted management.
Improved warehouse efficiency
AI boosts throughput and efficiency multi-fold within warehouses by automating movements thereby reducing reliance on human-driven activities. Computer-vision robots and process automation enhances volumes per shift while AI-optimized slotting and cluster picking minimizes travel distances and times. With AI-powered dynamic task assignments and exception handling based on changing priorities, workers focus on value-added tasks. Eliminating idle waits during shifts, AI improves equipment and workforce utilization by 30-40%. Further, predictive maintenance alerts on AI avoids disruptions from breakdowns. Overall these translate to lowered warehousing costs.
Better supply chain sustainability
AI substitutes carbon-intensive processes like physical audits with digitally driven automated validation and reconciliation exercises. Using optimization algorithms helps schedule electric vehicles, recommend renewable energy-powered warehousing locations as well as multi-modal shifts for transportation. AI-powered demand forecasting ensures minimal leftover inventory and stock imbalances mitigating embedded carbon footprints of procured but unutilized materials. Factoring sustainability parameters, AI routing designs supply chain networks and lanes optimally. By driving resource efficiency at an ecosystem level, AI delivers up to 40% reductions in carbon emissions and fosters green operations for businesses.
Optimized operations through simulation
AI proves instrumental in testing hypothetical operational scenarios without real disruptions through its digital twin and hyper-realistic simulation abilities. Experiments through AI-powered simulations help identify optimized policies for production, inventory, warehousing and transportation management upfront before actual deployment. It reduces risks through virtual analysis of various externalities and proactively suggests right course corrections. AI, therefore, empowers data-driven decisions with low experimentation costs. Overall it enhances competitiveness, resilience and future-readiness of technology-integrated supply chains.
Addressing AI in Supply Chain Challenges
While AI delivers ample benefits to modern supply chains, its adoption also brings challenges for organizations to address. Key risks involve lack of skills and expertise, sizable costs especially during initial proof-of-concept implementations as well as complex solution integrations. Data quality challenges also arise that AI models cannot compensate for. If unaddressed properly, these can defeat the very purpose. Let’s understand some critical challenges and suggested mitigation strategies.
Downtime for training
Transition to AI demands extensive training for equipping existing manpower with new skills as well as ramping-up capabilities to handle evolving systems. Although required, this slows business-as-usual activities and needs careful planning of cutovers. Strategies to mitigate impacts involve pilot implementations, evening/weekend training schedules along with documenting runbooks, and help resources for real-time support during transitions. Automated training through e-learning and gamified systems further streamlines skills transfers. Comprehensive change management preempts cultural roadblocks through education and incentives. Overall downtime impacts can reduce to 1-2% of total timelines through well- rounded preparations.
Startup costs
Large capital outlays on AI solutions, integration services, technologies and talent acquisitions impose financial burdens. While necessitated, return on these investments takes time. Addressing this involves staggered implementation through modular deliveries aligned with business priorities and quantifiable wins. Further, outsourcing non-core AI tasks to specialized partners helps stay lean and focus on core operations. Taking advantages of tax rebates, subsidies on green and digital innovations also softens costs. Demonstrating early Use cases of AI in Supply Chain for cross-functional buy-ins and securing budgetary commitment post-proofs further eases initial commitments. Proper funds allocation thus ensures rapid value realization.
Complex systems
Integrating AI with diverse legacy platforms across myriad touchpoints poses technical challenges. However, a modular microservices-based design segmented per business domains eases integration burdens. Establishing centralized data lakes and governance frameworks provides requisite oversight and controls. Leveraging open-source/plug-and-play components wherever possible along with low-code development kits streamlines customizations and scaling. Further, using specialized system integrators having domain expertise helps achieve holistic executions within timelines. Focus thus remains on extractable benefits over just technology integration complexities.
AI risks
There exist inherent risks around AI like inaccuracy, quality and reliability of training data affecting model outcomes. To counter, companies must institute governance controls involving 1) data quality checks 2) model monitoring mechanisms 3) change control procedures 4) fallback arrangements 5) version control and upgrades whenever necessitated. They must also factor risks at design stages themselves to engineer controls and protections. For example, implementing canary releases, sophisticated testing frameworks, debug switches and thorough documentation eases management and resolutions. An AI Centre of Excellence with capabilities on monitoring, audit as well as detection and response further strengthens risk mitigation posture of organizations.
Inaccuracy of data
As AI solely relies on available training information, any gaps or bias in underlying data leads to prejudiced outcomes. It is thus important to sanitize and enrich datasets in representation of true target populations. Strategies involve adding more contextual/demographic attributes, handling outliers/anomalies carefully as well as continual augmentation practices. Enlisting domain experts helps examine sufficiency from lenses of societal fairness, coverage and privacy preservation. Quality benchmarks along with version controls on data further assure AI systems function as intended over time. Overall, data integrity and evolving practices form center of successful model deployments.
Over Dependence on AI
While AI boosts efficiencies, human cognition faculties remain irreplaceable especially for complexities beyond current models. Over-reliance thus breeds risks from losing control and troubleshooting capabilities, reduced adaptiveness as well as single-point failures during outages. Mitigation focuses on empowering, not replacing existing expertise through cognitive augmentation. Core activities must stay driven by human experts aided, not supplanted by AI. Further, establishing contingencies like manual overrides, model fallbacks and AI specialists secures effective problem resolutions. Proper change management also prevents complete dependency mindsets enterprise-wide. AI hence plays an assisting, non-authority role in foreseeable future.
Security and privacy vulnerabilities
As AI systems process and retain immense volumes of sensitive commercial and personal information, associated risks around breaches escalate. Key concerns pertain to lack of access controls, unauthorized data exposure as well as model inversion attacks extracting information. Adopting principles of privacy by design, employing strong access management, regular security testing along with anonymizing sensitive fields helps address such exposures. AI systems must also be designed keeping regulations like GDPR in mind to inspire confidence among partners, customers and regulators alike. Overall, instituting robust security practices forms the basis to leverage AI innovations responsibly.
Turn AI Insights into Supply Chain Success – Book A 30 Minutes Free Consultation!
Conclusion
In conclusion, AI is fast emerging as the prime catalyst enabling disruption and scaling across modern supply chain ecosystems. By leveraging Machine Learning capabilities for predictive and prescriptive analytics, companies are optimizing operations significantly to achieve sustainability and build resilience amid changing business environments. While initial investments may seem substantial, tangible value delivered through automated and data-driven decision making provides strong returns justifying costs. With proper preparation and mitigation of risks, AI transforms supply chains from functional silos to strategic assets delivering competitive differentiators for businesses of all sizes. Its widespread adoption makes supply chain management an exciting frontier for the next generation of innovations.
How can A3Logics Help in Optimizing Artificial intelligence in Supply Chain
A3Logics is an Enterprise AI Development Company known for developing customized Enterprise AI solutions. With its deep expertise in AI for supply chain domain, A3Logics can help clients successfully optimize critical facets of their Operations with Artificial intelligence in supply chain. Some key ways include:
- Demand Forecasting and Planning: A3Logics leverages cutting-edge Machine Learning techniques to develop highly accurate Demand Forecasting models. This helps clients optimize Production Planning, reduce Inventory levels and better meet Client demands.
- Inventory Optimization: A3Logics’ AI-powered Inventory Optimization tools help determine ideal stocking levels factoring attributes like lead times, seasonality for items. This improves Turns, reduces Obsolescence and frees up Working Capital.
- Transportation Management and Route Optimization: With its AI-powered TMS and Route Optimization modules, A3Logics enhances Fleet Efficiency, lowers Fleet Expenses as well as shrinks Carbon Footprint through alternative Energy-efficient routes.
- Supplier Relationship Management: A3Logics’ AI-driven SRM suite enables risk-based Supplier Selection and dynamic e-Procurement. This results in optimized costs, on-time deliveries and sustainable sourcing.
- Warehouse Management: Pre-built and Customizable WMS solutions from A3Logics automate Processes like Yard Management, Picking using Computer Vision and Slotting through AI for maximizing Throughputs.
By leveraging its data scientists, engineers and delivery capabilities, A3Logics ensures end-to-end AI implementations are customized, cost-effective and help clients unlock tremendous value from their Supply Chain Investments. Its expertise in Logistics, Retail and CPG domains provide requisite domain knowledge for successful AI transformations.
A3Logics, top Enterprise AI Chatbot Development company, can be contacted to understand discrete AI Problems and discuss engagement models for delivering Business Results. To know more about other AI Development Companies and top applications of AI like predictive maintenance, Please explore A3Logics website.
FAQs
How does AI improve demand forecasting in the supply chain?
AI improves demand forecasting in supply chains by leveraging machine learning algorithms. These algorithms analyze historical sales patterns, market trends, pricing, customer demographics and other factors to generate highly accurate demand forecasts. AI considers various external and internal attributes to forecast personalized needs upfront. By predicting demand precisely at a micro-level for each product and location, AI helps optimize inventory levels, meet customer demands better as well as reduce forecast errors significantly. This in turn enhances fill rates, reduces wastages and improves overall supply chain efficiencies.
What role does AI play in warehouse automation?
AI plays a vital role in automating warehousing processes. Using computer vision, robots, drones and other automation technologies powered by AI, processes like goods to person picking, put away, inventory audits etc are performed autonomously. AI powers automated sorting, routing of goods as well as dynamic task allocation for warehouse workers. This enhances throughputs multi-fold while improving safety. AI driven slotting, cluster picking and predictive maintenance further optimize asset utilization. Overall, AI eliminates manual work, improves productivity within warehouses and reduces associated costs substantially.
How does AI contribute to transportation and route optimization?
AI contributes hugely to optimizing transportation networks. By analyzing historical journeys, assessing traffic patterns and stochastic variables in real-time, AI powered algorithms generate thousands of optimized route plans for vehicles. This helps choose energy-efficient routes, synchronize loading dock arrivals as well as adjust dynamically in case of exceptions like road diversions. AI routes help lower fuel spends, CO2 emissions and expedite deliveries by avoiding delays. It also balances workloads efficiently across fleets and assets to maximize their usage leading to higher savings.
Can AI assist in supplier selection and management?
Yes, AI can greatly assist in supplier selection and management. AI analyzes supplier past performance, current capacities, risks, quality, costs and other weighted attributes objectively to rank and recommend best fitting partners transparently. It also facilitates two-way digital engagements, powers automated procure-to-pay cycles and tracks order progress end-to-end. AI enhances supply chain visibility, triggers alerts for issues and helps negotiate optimized contracts factors like sustainability. Overall, AI enables data-driven, cost-effective and sustainable sourcing decisions for businesses.
What is the benefit of predictive maintenance in supply chains?
Predictive maintenance powered by AI and machine learning delivers substantial benefits to supply chain equipment including vehicles, conveyors, cranes etc. By continuously monitoring asset health parameters, AI predicts component failures well in advance through anomaly detection. This helps schedule planned servicing without disturbing operations. AI avoids unpredictable breakdowns, associated repair costs as well as penalties of shipment delays. It enhances asset reliability considerably by 30-40%. AI driven predictive maintenance is thus transforming uptime, utilization and overall equipment effectiveness across supply chain infrastructure vastly. For more information you can always connect with the experts providing AI Development Services at A3Logics.